A hybrid Finite Elemente (FE) and Artificial Intelligence (AI)-driven approach for accurate brain shift prediction in image-guided neurosurgery - digibrAIn
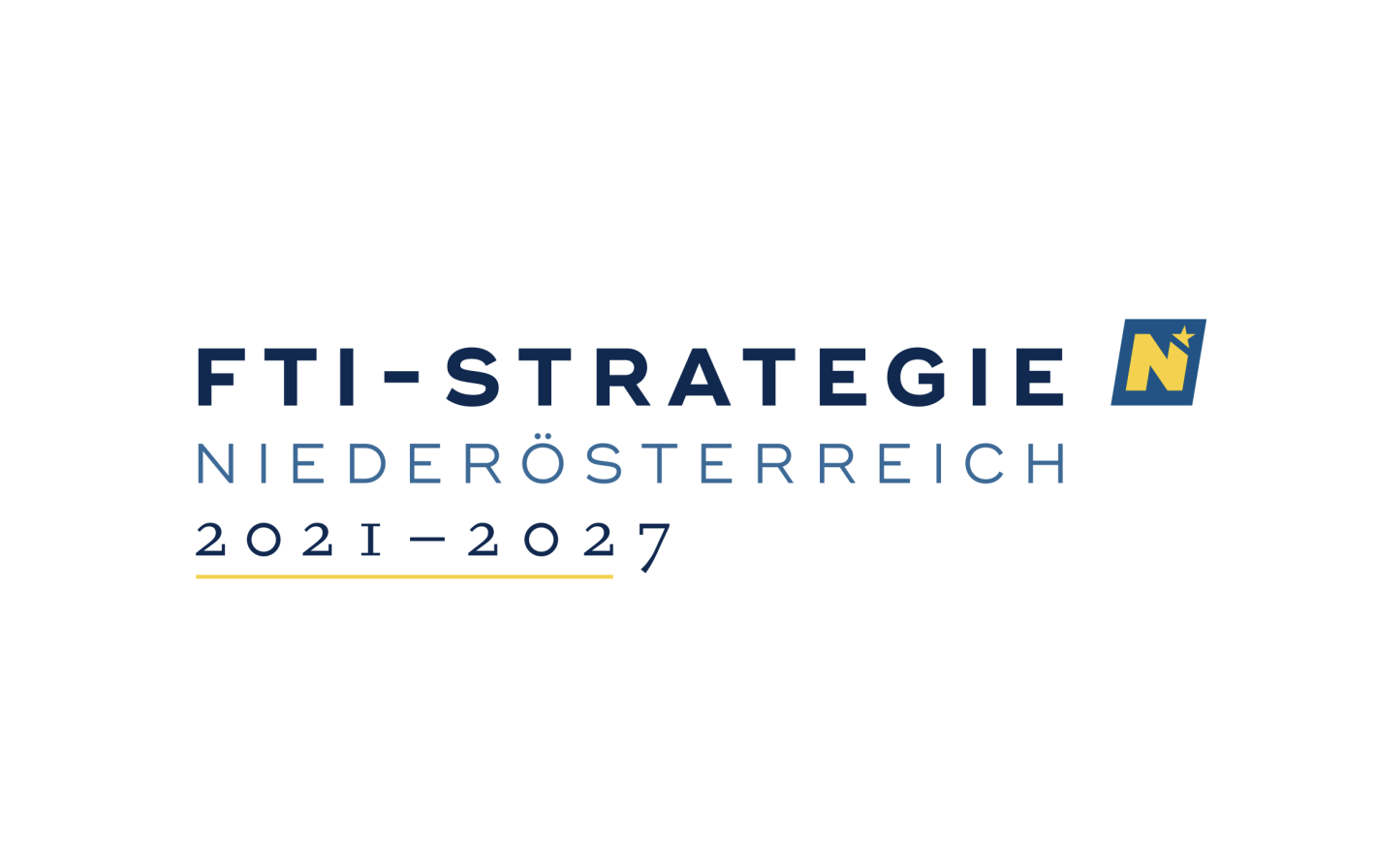
Lead partner:
ACMIT - Austrian Center for Medical Innovation and Technology
Scientific management:
Gernot Kronreif
Additional participating institutions:
Danube Private University
Technische Universität Wien
Field(s) of action:
Health and nutrition
Scientific discipline(s):
2060 - Medizintechnik (55 %)
1020 - Informatik (45 %)
Funding tool: Basic research projects
Project-ID: FTI24-G-024
Project start: 01. April 2025
Project end: 31. März 2028
Runtime: 36 months / ongoing
Funding amount: € 360.000,00
Brief summary:
In brain tumor surgeries, image-guided neurosurgical systems (or neuro-navigation systems, respectively) help
neurosurgeons to locate tumors by aligning preoperative image data with the patient's coordinate system. However,
brain deformation, aka brain shift, can lead to misalignment between estimated and actual tumor positions and
increase the risk of brain damage. Existing methods for the compensation of brain shift often involve additional
intra-operative imaging (MRI or ultrasound) or are initiated by manual landmark registration, both of which disrupt the
surgical workflow.
In the digibrAIn project, we propose a novel computational approach that uses intraoperative image data from the
stereomicroscope after opening the skull (craniotomy) and the dura mater to perform automatic, AI-based non-rigid
registration without interference with the established clinical workflow. Our framework utilizes advanced deep learning
techniques for non-rigid 3D point cloud registration, combined with synthetic training data generated by Finite Element
Method (FEM) to realistically represent potential brain structure deformations. Additionally, it takes advantage of
transfer learning to enable the time-consuming initial FEM simulations and AI model training based on the diagnostics
MRI weeks before surgery, and then upgrade the model based on the most recent information from a planning MRI the
day before surgery in a fast and efficient way. As part of the project, the proposed approach will be verified using a
novel instrumented brain phantom by comparing measured and predicted brain shifts.
This framework has the potential to accurately predict real-time brain shift, support the planning of optimal surgery
trajectories and guide surgeons by providing updated information of tumor location and critical brain structures without
disrupting the workflow. Ultimately, our approach aims to enhance surgical quality, improve patient safety, and
positively impact patient’s quality of life.
Keywords:
neurosurgery, brain tumor, digital brain shift tracking, image-guided, artificial intelligence, deep learning, transfer learning, finite element method, brain phantom