Anwendung von Active Machine Learning zur automatischen Schreibererkennung in Manuskripten des 12. Jahrhunderts
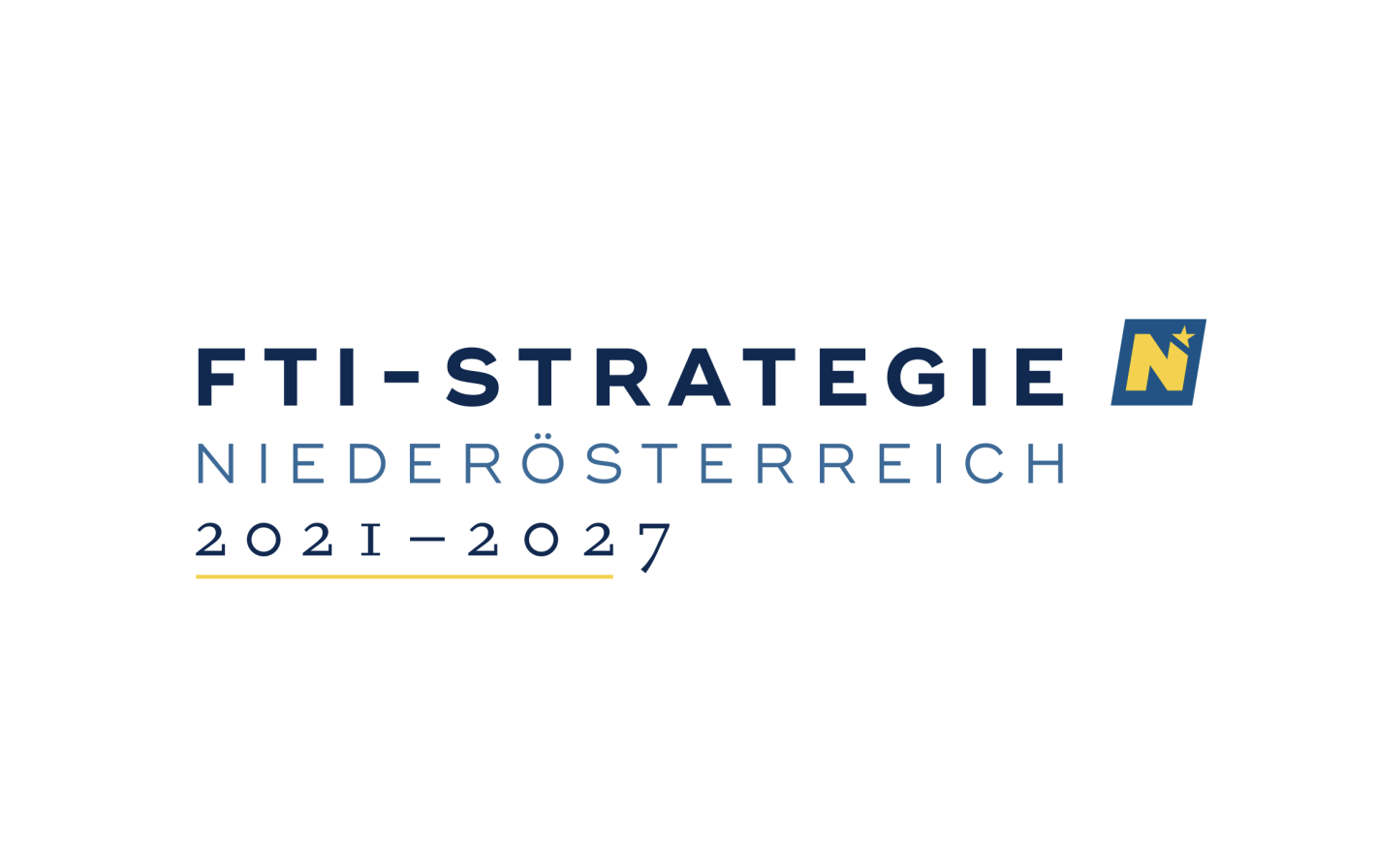
Lead partner:
Fachhochschule St. Pölten
Scientific management:
Markus Seidl
Additional participating institutions:
Stift Klosterneuburg
Research field:
Sammlungen Niederösterreich
Funding tool: Basic research projects
Project-ID: FTI18-004
Project start: 01. März 2020
Project end: will follow
Runtime: 36 months / ongoing
Funding amount: € 199.700,00
Brief summary:
Today’s Lower Austria has an outstanding international position due to the large number of still active monasteries, whose tradition is unbroken since their foundation in the Middle Ages. In the last third of the 12th century the monks and canons in the monasteries began to systematically collect books. By the turn of the century, around the year 1200, large libraries were built and most of them are still preserved today. About 120 manuscripts from this period can be found in Heiligenkreuz, more than 130 in Zwettl and almost 120 codices in the monastery library Klosterneuburg.
The main goal of our work is to enable a better understanding of monastic scriptoria in high mediaeval Austria through a broader knowledge about the scribes that have been working in these early centers of knowledge. However, there is no evidence about the number of scribes and whether they worked in only one cloister or moved around between the monasteries. A way to determine these factors is by analysing the styles of writing with the goal to identify different scribes in a large number of manuscripts by inherent stylistic characteristics of their writings. Consequently, this allows to deduct the whereabouts of the scribes and the organisation of the scriptoria.
The classic way of analysis by single experts is a tedious and time-consuming process. This process involves the risk, that the results are not fully valid as these are generated subjectively by individual impressions. There are first approaches to support the identification of mediaeval writing hands by machine learning. However, these are not usable for large corpora.
The main challenge is the lack of extensive ground truth which poses a chicken-and-egg problem. In an interdisciplinary project involving historians and computer scientists, we are proposing an active machine learning approach that specifically involves human experts to support the machine learner and thus enables a time efficient scribes labelling for large corpora.